Apr 7, 2025
Apr 7, 2025
In the Heat of the Battery: Heat Generation & Thermal Modeling
In the Heat of the Battery: Heat Generation & Thermal Modeling

In our previous post, we explored internal resistance and how it affects power output, efficiency, and—critically—heat generation. When a battery operates, not all of the energy goes into powering devices; some is inevitably lost as heat due to internal resistance and other electrochemical processes. Managing this heat is essential, especially in large-format cells used in electric vehicles or grid storage, where temperature fluctuations can impact performance, lifespan, and safety. This is where thermal models come into play.
Every battery generates heat during operation. The main sources of heat include Ohmic losses, reaction overpotentials, and concentration gradients within the electrodes and electrolyte. In small cells or at low currents, the generated heat may dissipate naturally. However, in high-power or large-capacity applications, heat can accumulate, leading to temperature rises that affect battery performance and accelerate degradation. In extreme cases, excessive heat can trigger thermal runaway—a dangerous, self-reinforcing cycle of overheating.
It’s crucial to accurately model how battery temperature evolves over time, and that’s precisely what thermal models do. These models are coupled with electrochemical models, such as the Doyle-Fuller-Newman (DFN) model: the electrochemical processes dictate how much heat is generated, while the battery temperature affects transport properties inside the cell. This coupling makes the models significantly more complex but crucial for accurate predictions.
At the core of thermal modeling is the heat equation, which governs the conservation of energy in the system. However, there are different versions we can use in thermal modelling of batteries:
Lumped Thermal Models: These treat the entire battery as having a uniform temperature. While simpler and computationally efficient, they are most suitable for systems where temperature gradients are minimal or for real-time battery management systems (BMS) that require fast calculations.
Spatially Distributed Models: These account for temperature variations within the battery. Depending on the desired level of resolution, some models capture temperature differences across the current collector or cell thickness, while others provide detailed variations within each battery layer. This approach is essential for large-format cells or high-power applications, where internal temperature gradients can significantly impact performance and safety.
Choosing between these approaches depends on the specific application and the required level of accuracy. In practice, many battery management systems use simplified models for real-time control, while more detailed models are employed for design and analysis.
Temperature control isn’t just about efficiency—it directly influences battery aging. Elevated temperatures accelerate chemical degradation processes, leading to capacity fade and increased internal resistance. And that brings us to our next topic: battery degradation and State of Health (SoH).
In our previous post, we explored internal resistance and how it affects power output, efficiency, and—critically—heat generation. When a battery operates, not all of the energy goes into powering devices; some is inevitably lost as heat due to internal resistance and other electrochemical processes. Managing this heat is essential, especially in large-format cells used in electric vehicles or grid storage, where temperature fluctuations can impact performance, lifespan, and safety. This is where thermal models come into play.
Every battery generates heat during operation. The main sources of heat include Ohmic losses, reaction overpotentials, and concentration gradients within the electrodes and electrolyte. In small cells or at low currents, the generated heat may dissipate naturally. However, in high-power or large-capacity applications, heat can accumulate, leading to temperature rises that affect battery performance and accelerate degradation. In extreme cases, excessive heat can trigger thermal runaway—a dangerous, self-reinforcing cycle of overheating.
It’s crucial to accurately model how battery temperature evolves over time, and that’s precisely what thermal models do. These models are coupled with electrochemical models, such as the Doyle-Fuller-Newman (DFN) model: the electrochemical processes dictate how much heat is generated, while the battery temperature affects transport properties inside the cell. This coupling makes the models significantly more complex but crucial for accurate predictions.
At the core of thermal modeling is the heat equation, which governs the conservation of energy in the system. However, there are different versions we can use in thermal modelling of batteries:
Lumped Thermal Models: These treat the entire battery as having a uniform temperature. While simpler and computationally efficient, they are most suitable for systems where temperature gradients are minimal or for real-time battery management systems (BMS) that require fast calculations.
Spatially Distributed Models: These account for temperature variations within the battery. Depending on the desired level of resolution, some models capture temperature differences across the current collector or cell thickness, while others provide detailed variations within each battery layer. This approach is essential for large-format cells or high-power applications, where internal temperature gradients can significantly impact performance and safety.
Choosing between these approaches depends on the specific application and the required level of accuracy. In practice, many battery management systems use simplified models for real-time control, while more detailed models are employed for design and analysis.
Temperature control isn’t just about efficiency—it directly influences battery aging. Elevated temperatures accelerate chemical degradation processes, leading to capacity fade and increased internal resistance. And that brings us to our next topic: battery degradation and State of Health (SoH).
In our previous post, we explored internal resistance and how it affects power output, efficiency, and—critically—heat generation. When a battery operates, not all of the energy goes into powering devices; some is inevitably lost as heat due to internal resistance and other electrochemical processes. Managing this heat is essential, especially in large-format cells used in electric vehicles or grid storage, where temperature fluctuations can impact performance, lifespan, and safety. This is where thermal models come into play.
Every battery generates heat during operation. The main sources of heat include Ohmic losses, reaction overpotentials, and concentration gradients within the electrodes and electrolyte. In small cells or at low currents, the generated heat may dissipate naturally. However, in high-power or large-capacity applications, heat can accumulate, leading to temperature rises that affect battery performance and accelerate degradation. In extreme cases, excessive heat can trigger thermal runaway—a dangerous, self-reinforcing cycle of overheating.
It’s crucial to accurately model how battery temperature evolves over time, and that’s precisely what thermal models do. These models are coupled with electrochemical models, such as the Doyle-Fuller-Newman (DFN) model: the electrochemical processes dictate how much heat is generated, while the battery temperature affects transport properties inside the cell. This coupling makes the models significantly more complex but crucial for accurate predictions.
At the core of thermal modeling is the heat equation, which governs the conservation of energy in the system. However, there are different versions we can use in thermal modelling of batteries:
Lumped Thermal Models: These treat the entire battery as having a uniform temperature. While simpler and computationally efficient, they are most suitable for systems where temperature gradients are minimal or for real-time battery management systems (BMS) that require fast calculations.
Spatially Distributed Models: These account for temperature variations within the battery. Depending on the desired level of resolution, some models capture temperature differences across the current collector or cell thickness, while others provide detailed variations within each battery layer. This approach is essential for large-format cells or high-power applications, where internal temperature gradients can significantly impact performance and safety.
Choosing between these approaches depends on the specific application and the required level of accuracy. In practice, many battery management systems use simplified models for real-time control, while more detailed models are employed for design and analysis.
Temperature control isn’t just about efficiency—it directly influences battery aging. Elevated temperatures accelerate chemical degradation processes, leading to capacity fade and increased internal resistance. And that brings us to our next topic: battery degradation and State of Health (SoH).
In our previous post, we explored internal resistance and how it affects power output, efficiency, and—critically—heat generation. When a battery operates, not all of the energy goes into powering devices; some is inevitably lost as heat due to internal resistance and other electrochemical processes. Managing this heat is essential, especially in large-format cells used in electric vehicles or grid storage, where temperature fluctuations can impact performance, lifespan, and safety. This is where thermal models come into play.
Every battery generates heat during operation. The main sources of heat include Ohmic losses, reaction overpotentials, and concentration gradients within the electrodes and electrolyte. In small cells or at low currents, the generated heat may dissipate naturally. However, in high-power or large-capacity applications, heat can accumulate, leading to temperature rises that affect battery performance and accelerate degradation. In extreme cases, excessive heat can trigger thermal runaway—a dangerous, self-reinforcing cycle of overheating.
It’s crucial to accurately model how battery temperature evolves over time, and that’s precisely what thermal models do. These models are coupled with electrochemical models, such as the Doyle-Fuller-Newman (DFN) model: the electrochemical processes dictate how much heat is generated, while the battery temperature affects transport properties inside the cell. This coupling makes the models significantly more complex but crucial for accurate predictions.
At the core of thermal modeling is the heat equation, which governs the conservation of energy in the system. However, there are different versions we can use in thermal modelling of batteries:
Lumped Thermal Models: These treat the entire battery as having a uniform temperature. While simpler and computationally efficient, they are most suitable for systems where temperature gradients are minimal or for real-time battery management systems (BMS) that require fast calculations.
Spatially Distributed Models: These account for temperature variations within the battery. Depending on the desired level of resolution, some models capture temperature differences across the current collector or cell thickness, while others provide detailed variations within each battery layer. This approach is essential for large-format cells or high-power applications, where internal temperature gradients can significantly impact performance and safety.
Choosing between these approaches depends on the specific application and the required level of accuracy. In practice, many battery management systems use simplified models for real-time control, while more detailed models are employed for design and analysis.
Temperature control isn’t just about efficiency—it directly influences battery aging. Elevated temperatures accelerate chemical degradation processes, leading to capacity fade and increased internal resistance. And that brings us to our next topic: battery degradation and State of Health (SoH).
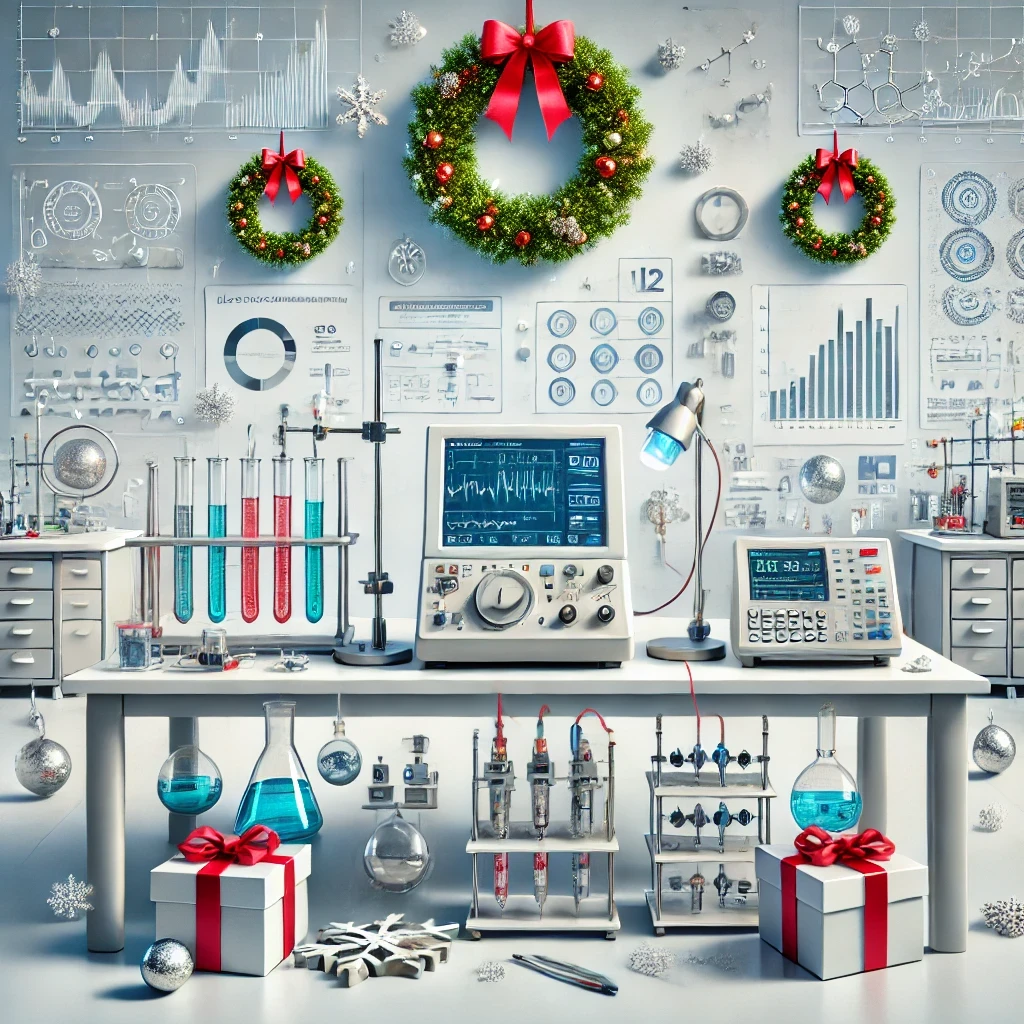
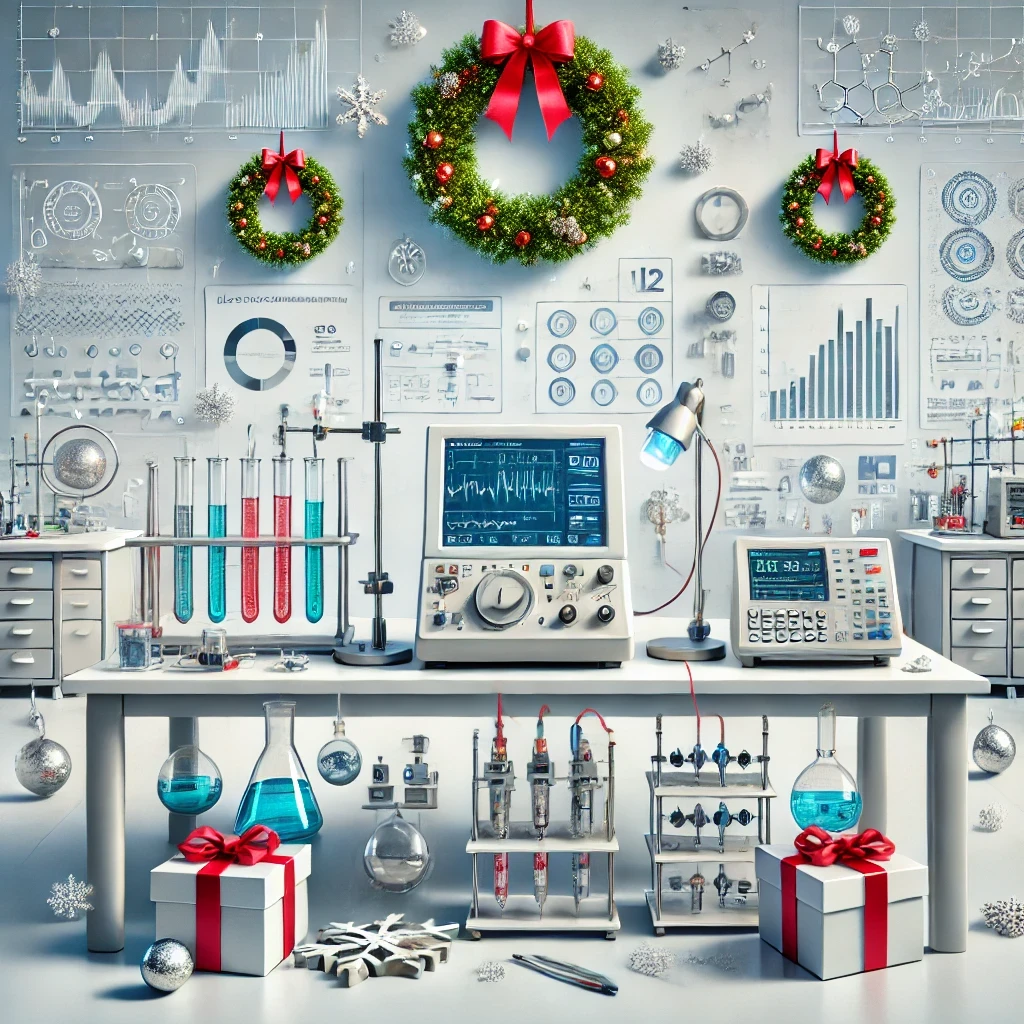
12 Days of Electrochemical Testing
To celebrate the holiday season and the re-release of Ionworks Studio, we featured "12 (business) days of electrochemical testing". Each day we pick a test, give a little bit of information about it, and show you how to run it in Ionworks. 🔋 🎄
Dec 17, 2024
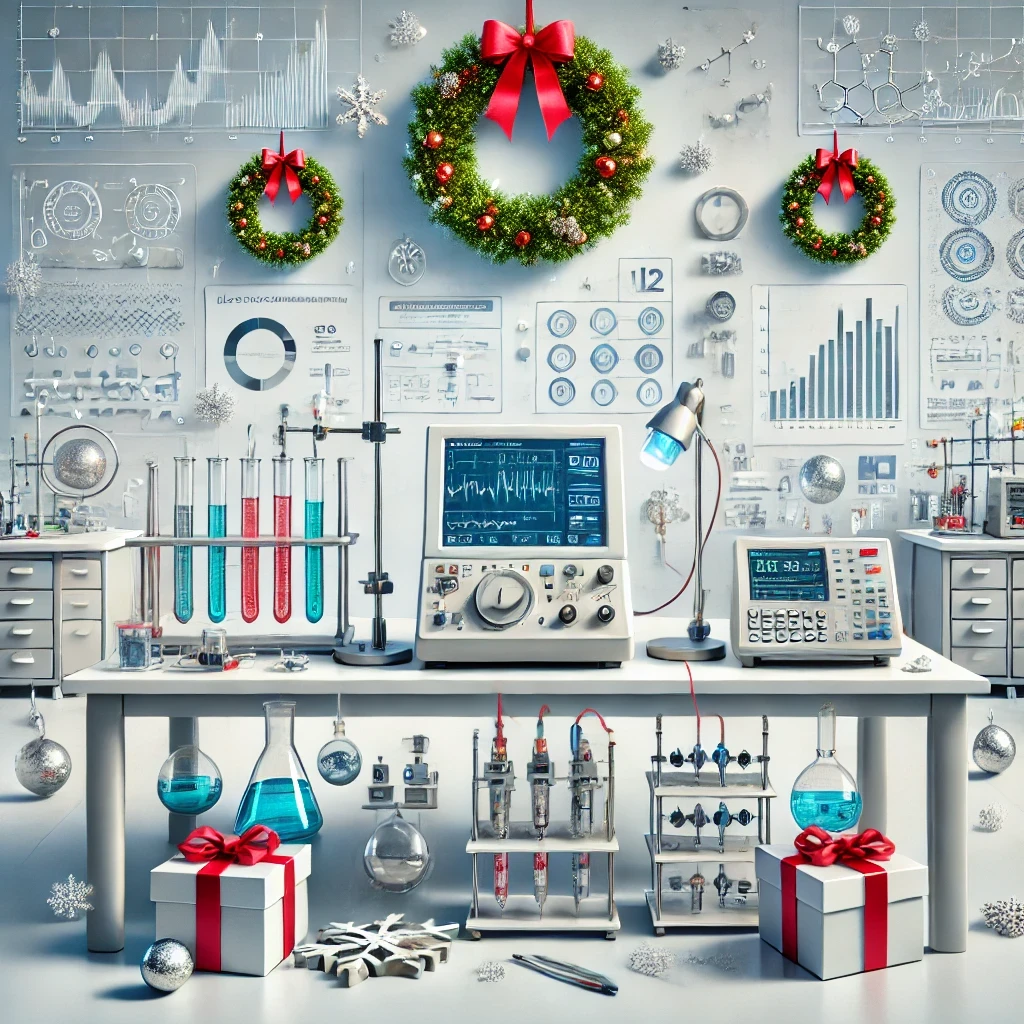
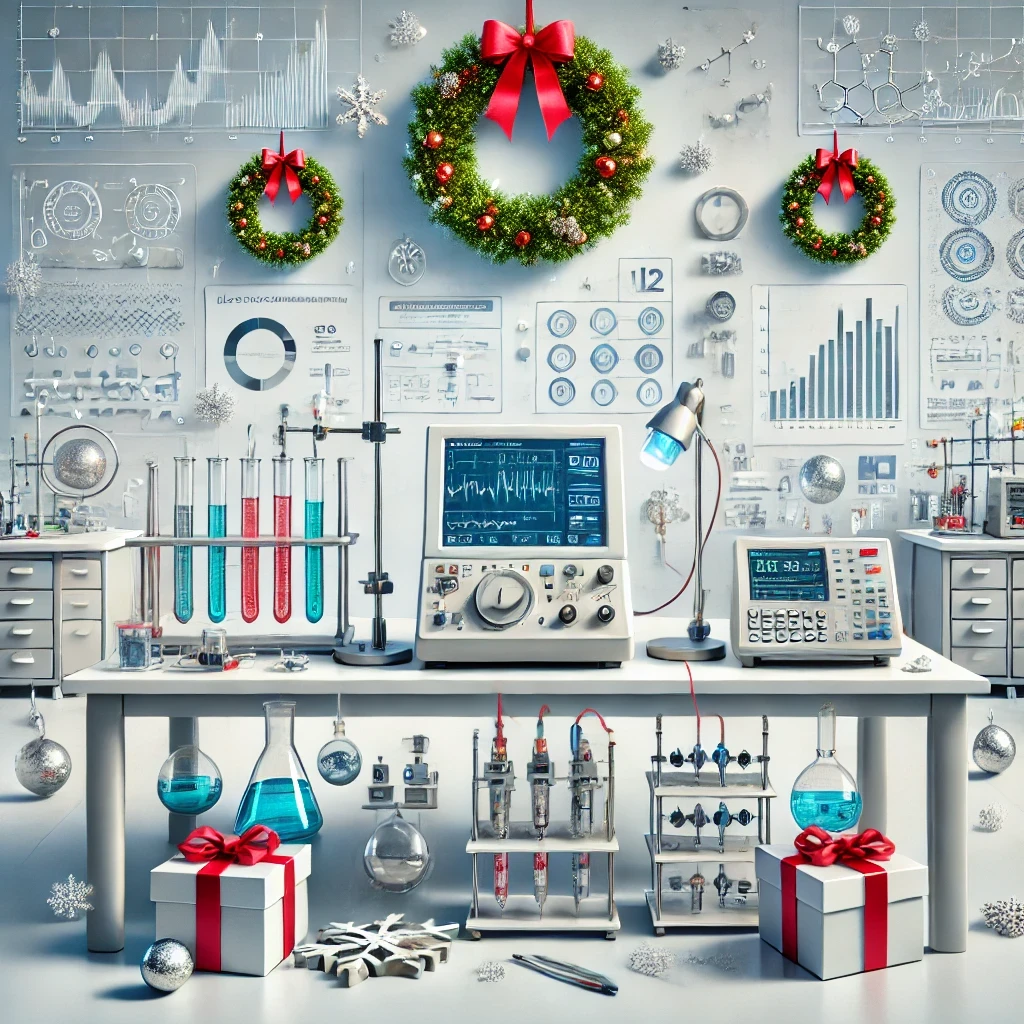
12 Days of Electrochemical Testing
To celebrate the holiday season and the re-release of Ionworks Studio, we featured "12 (business) days of electrochemical testing". Each day we pick a test, give a little bit of information about it, and show you how to run it in Ionworks. 🔋 🎄
Dec 17, 2024
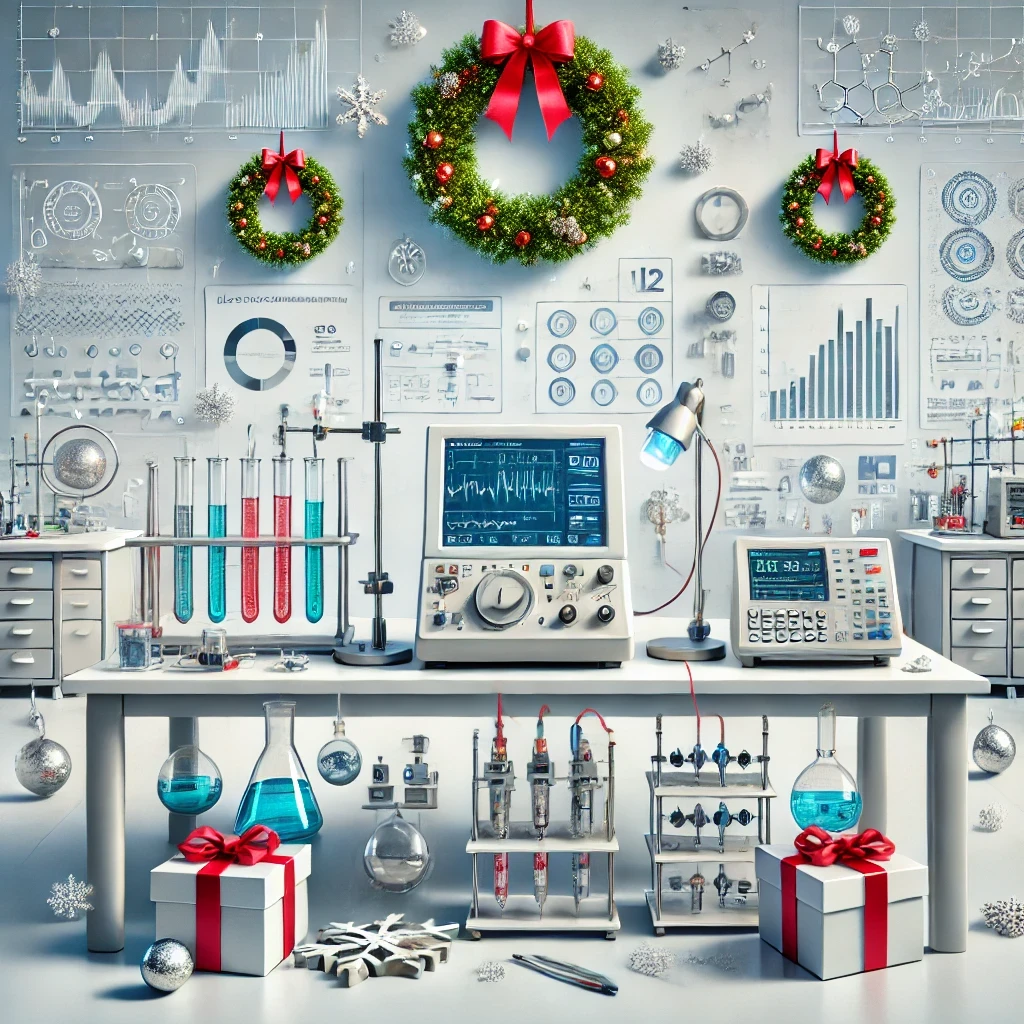
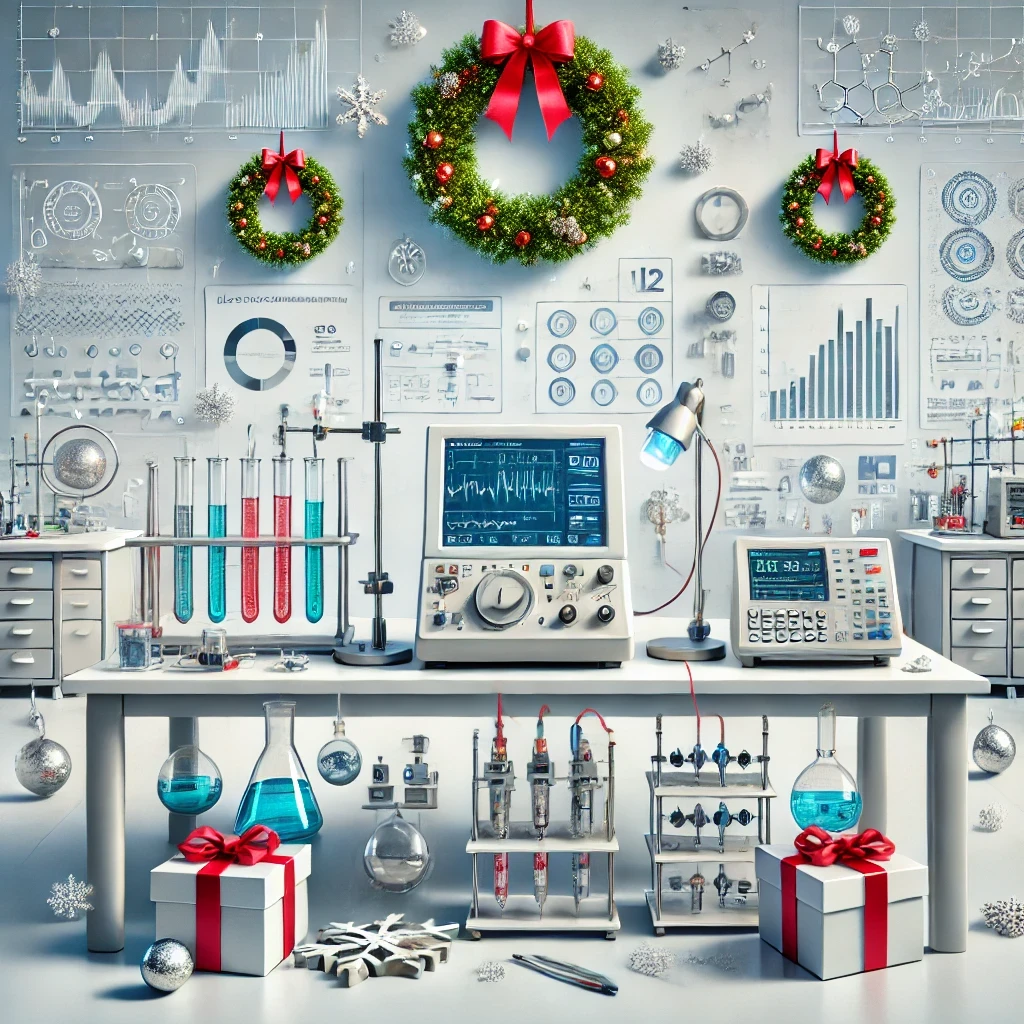
12 Days of Electrochemical Testing
To celebrate the holiday season and the re-release of Ionworks Studio, we featured "12 (business) days of electrochemical testing". Each day we pick a test, give a little bit of information about it, and show you how to run it in Ionworks. 🔋 🎄
Dec 17, 2024
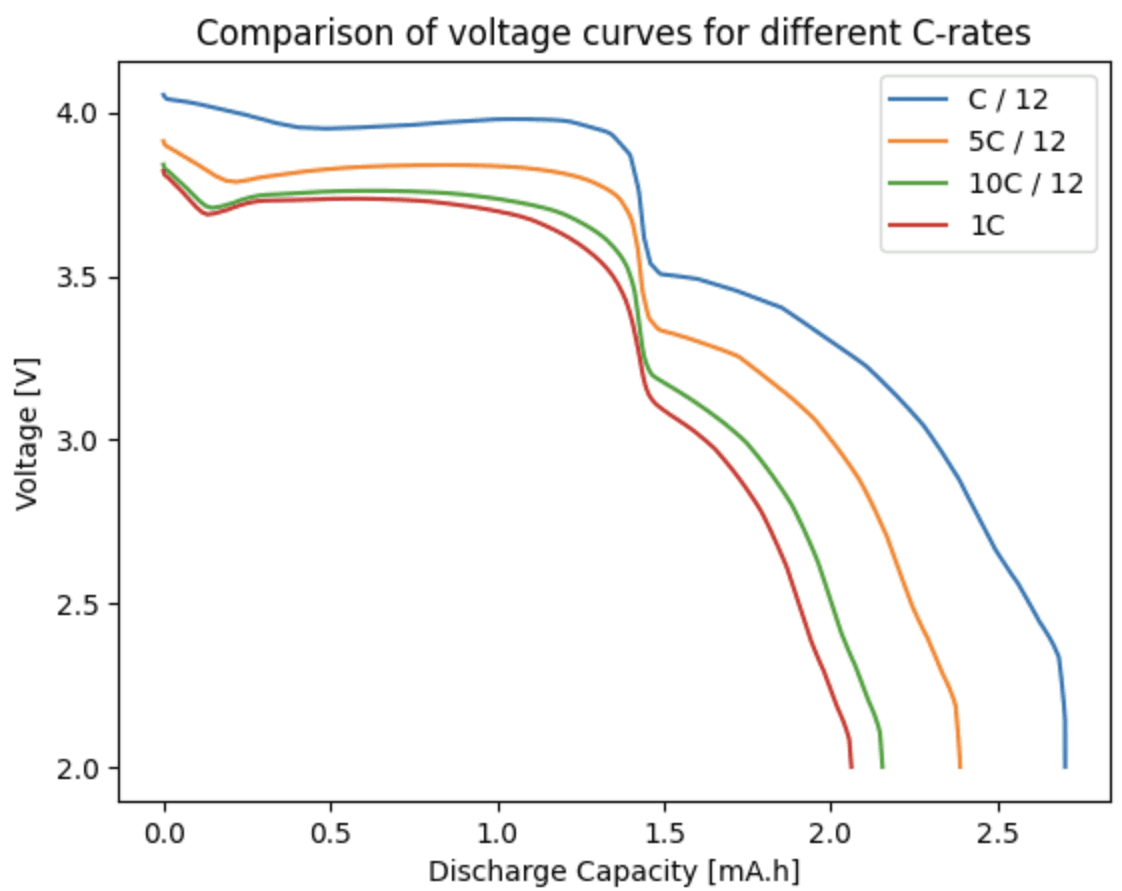
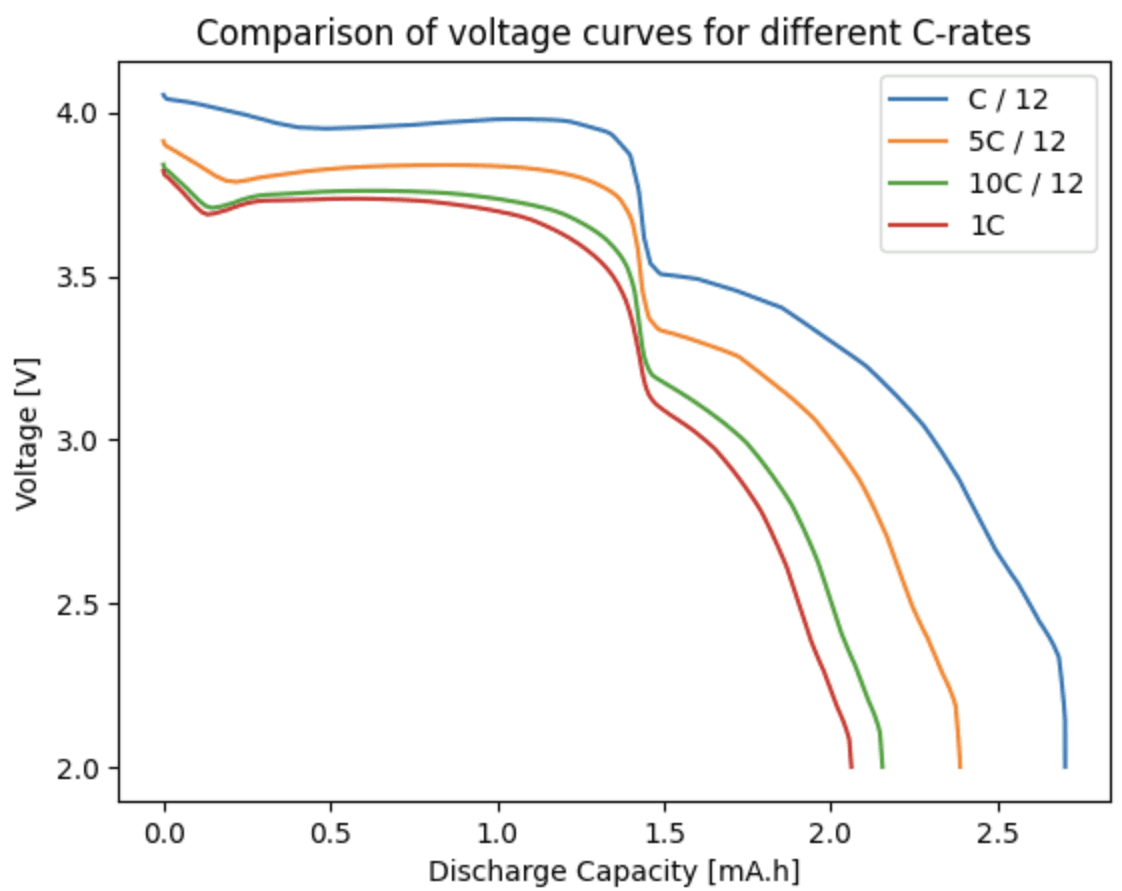
Sodium Ion Battery Model now available in PyBaMM!
This blog post explores the history of SIBs, the intricacies of the new PyBaMM model, and the exciting possibilities it unlocks for the future of energy storage.
Nov 12, 2024
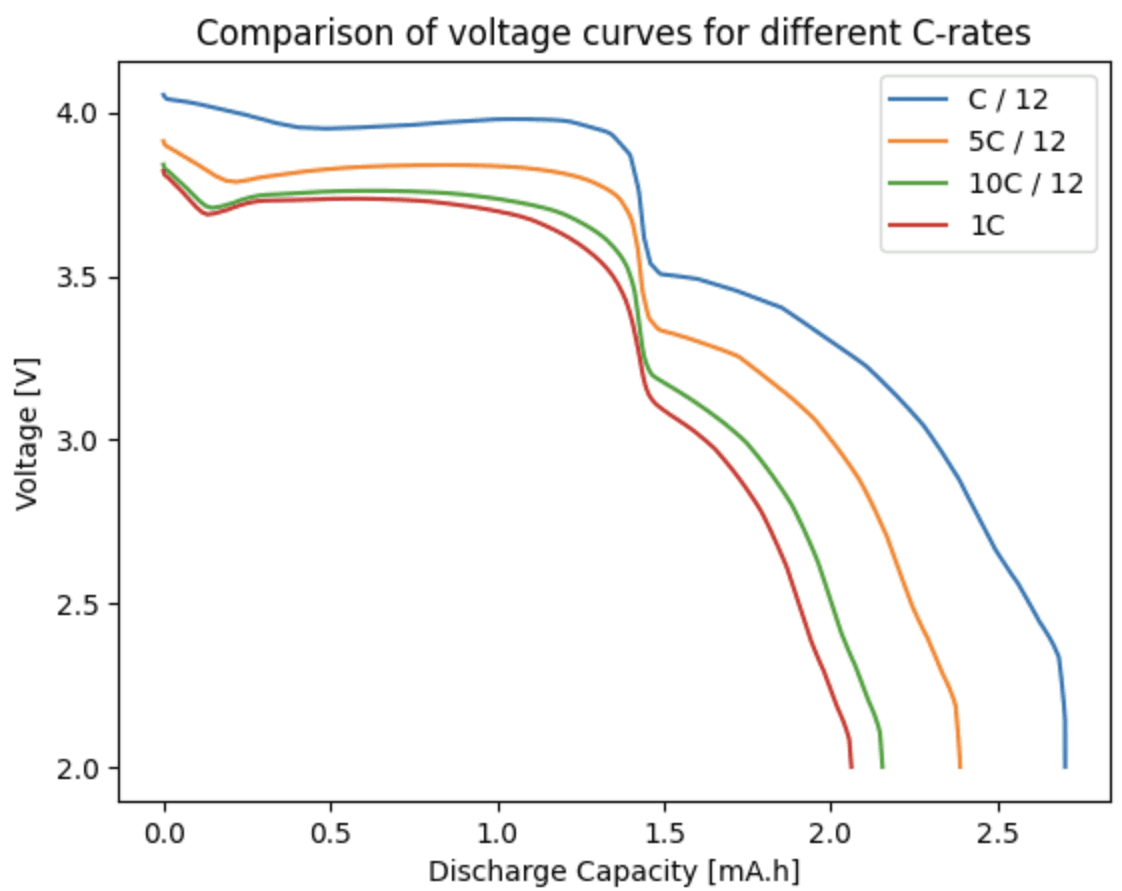
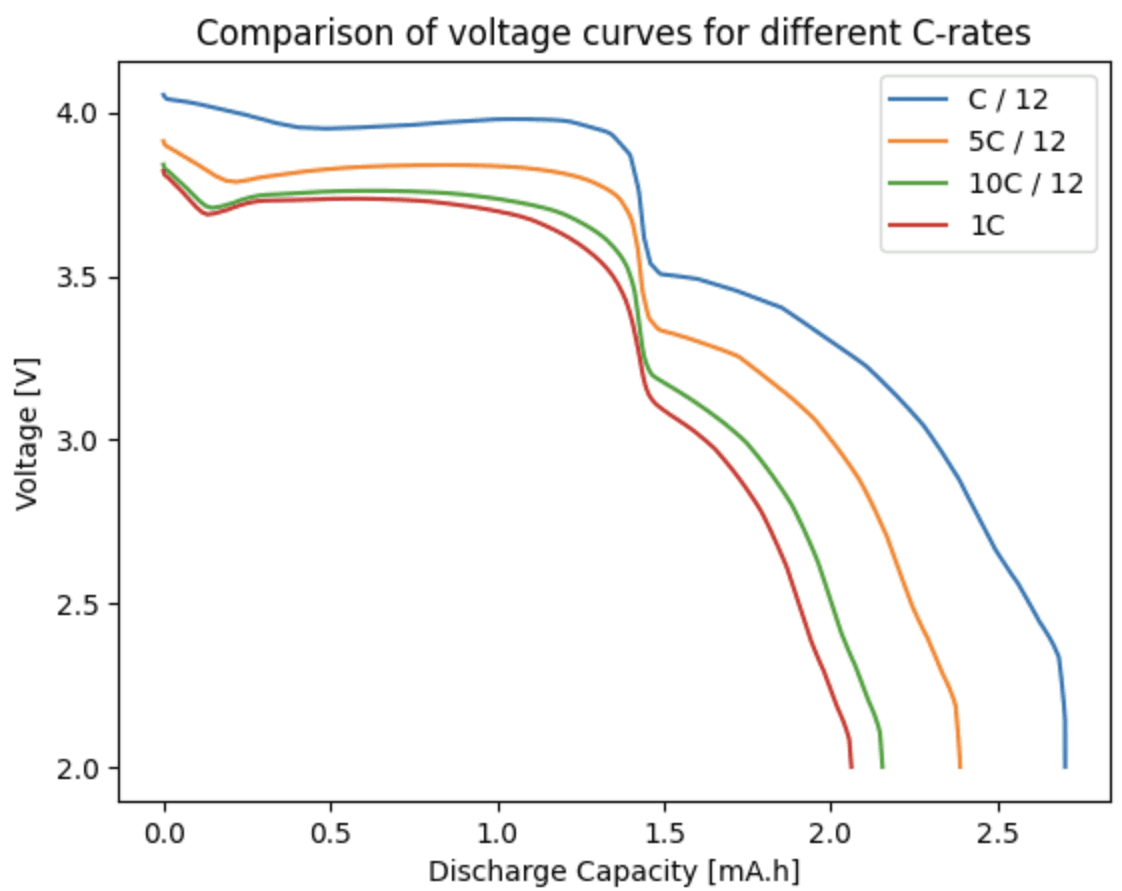
Sodium Ion Battery Model now available in PyBaMM!
This blog post explores the history of SIBs, the intricacies of the new PyBaMM model, and the exciting possibilities it unlocks for the future of energy storage.
Nov 12, 2024
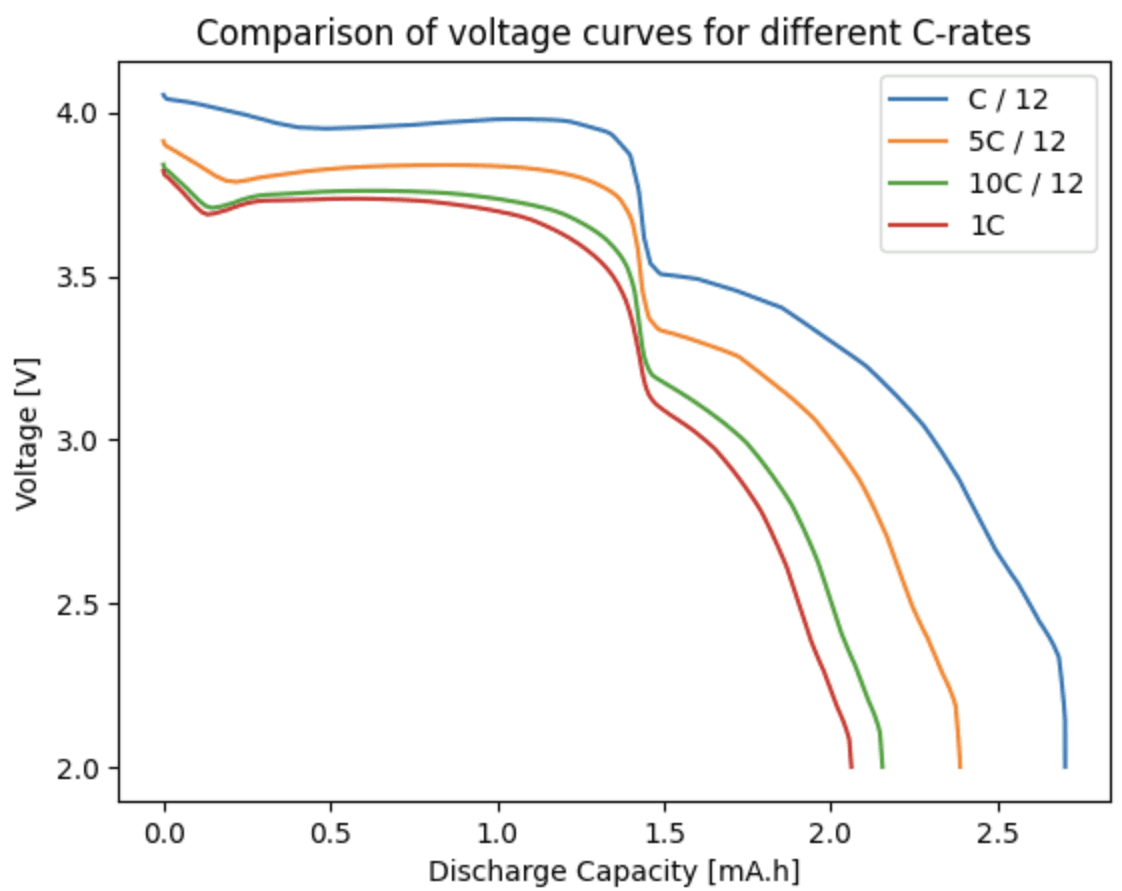
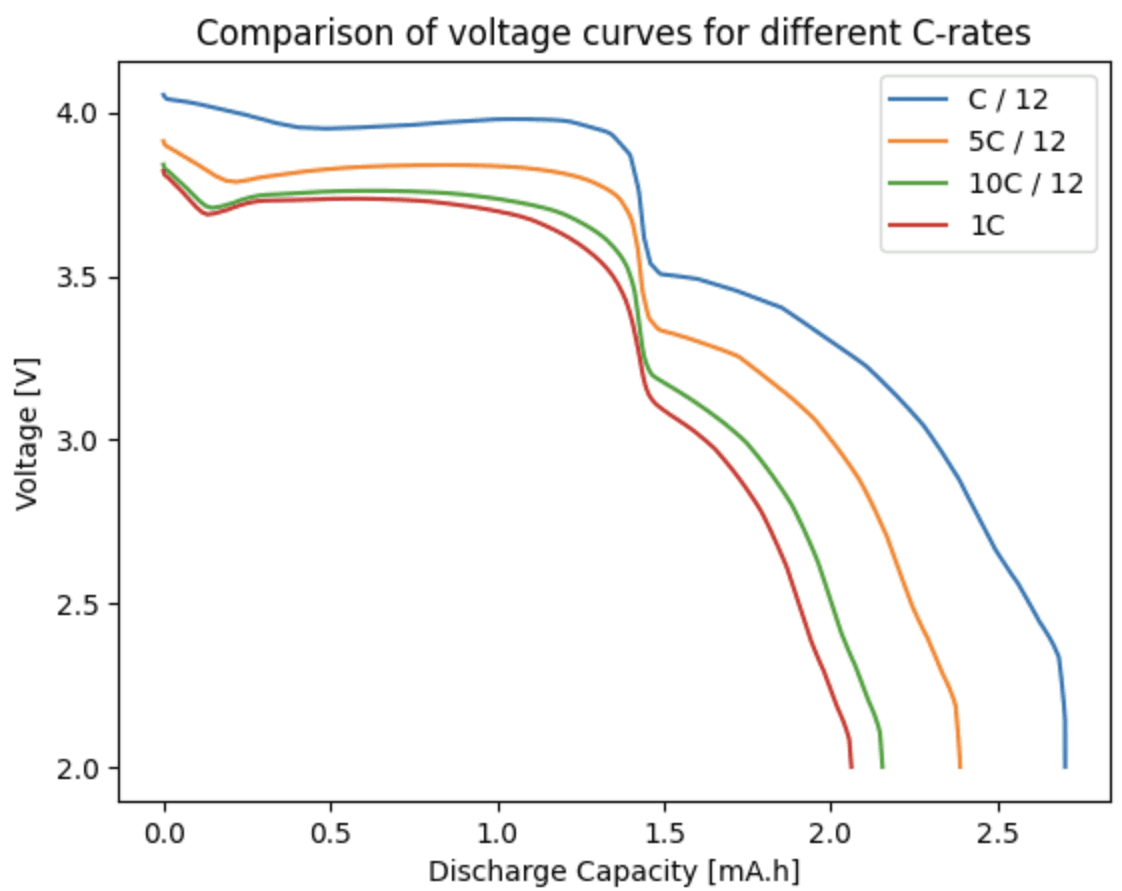
Sodium Ion Battery Model now available in PyBaMM!
This blog post explores the history of SIBs, the intricacies of the new PyBaMM model, and the exciting possibilities it unlocks for the future of energy storage.
Nov 12, 2024
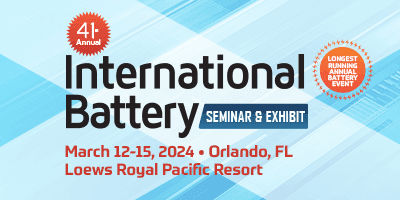
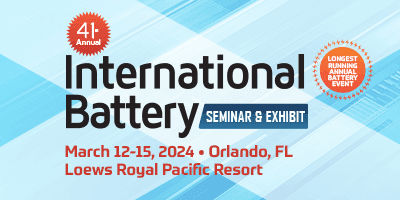
Ionworks Presents at International Battery Seminar
Ionworks CEO Valentin Sulzer presents at the International Battery Seminar in Florida
Mar 12, 2024
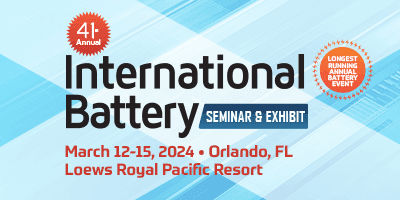
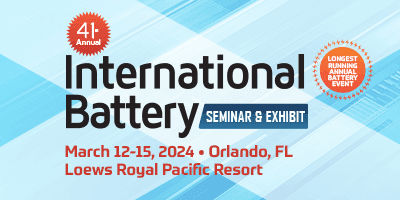
Ionworks Presents at International Battery Seminar
Ionworks CEO Valentin Sulzer presents at the International Battery Seminar in Florida
Mar 12, 2024
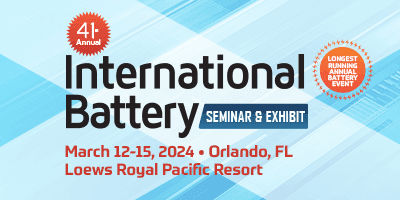
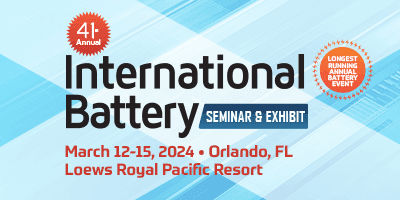
Ionworks Presents at International Battery Seminar
Ionworks CEO Valentin Sulzer presents at the International Battery Seminar in Florida
Mar 12, 2024
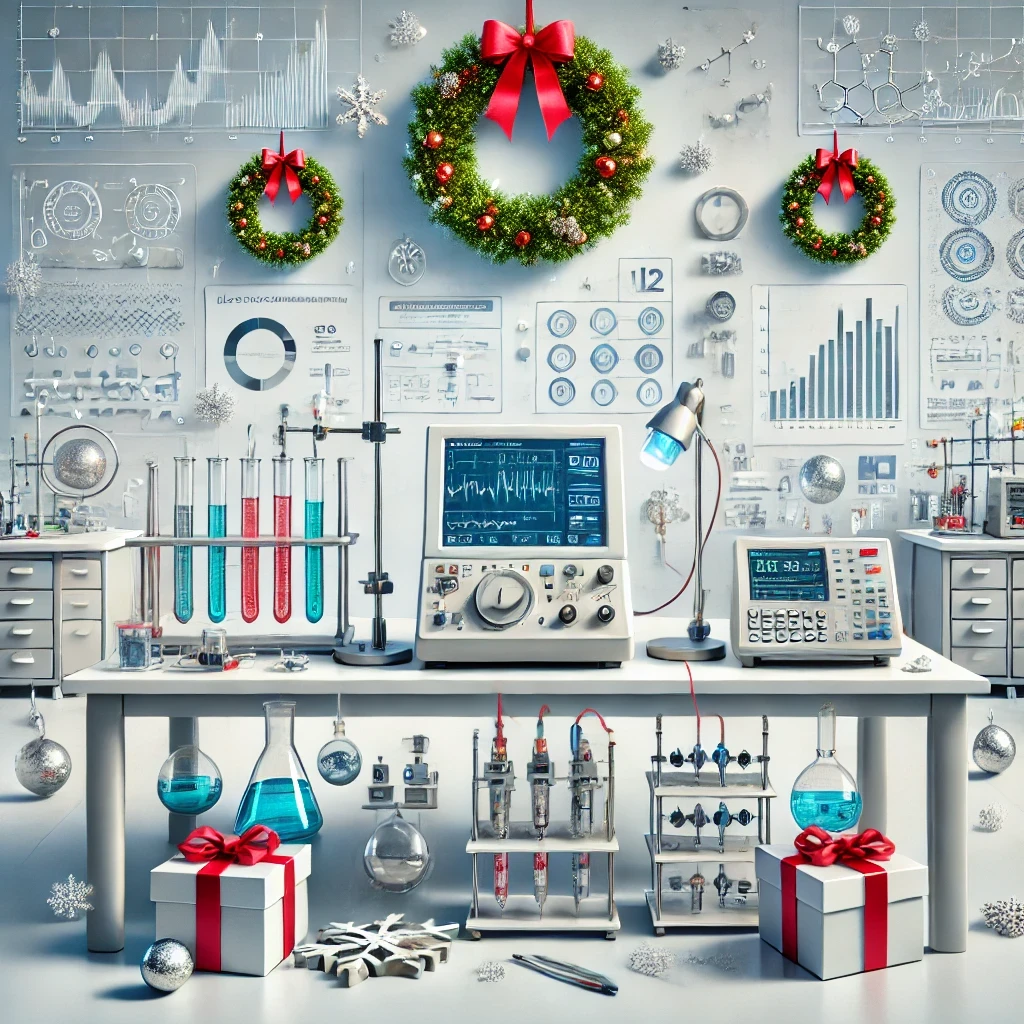
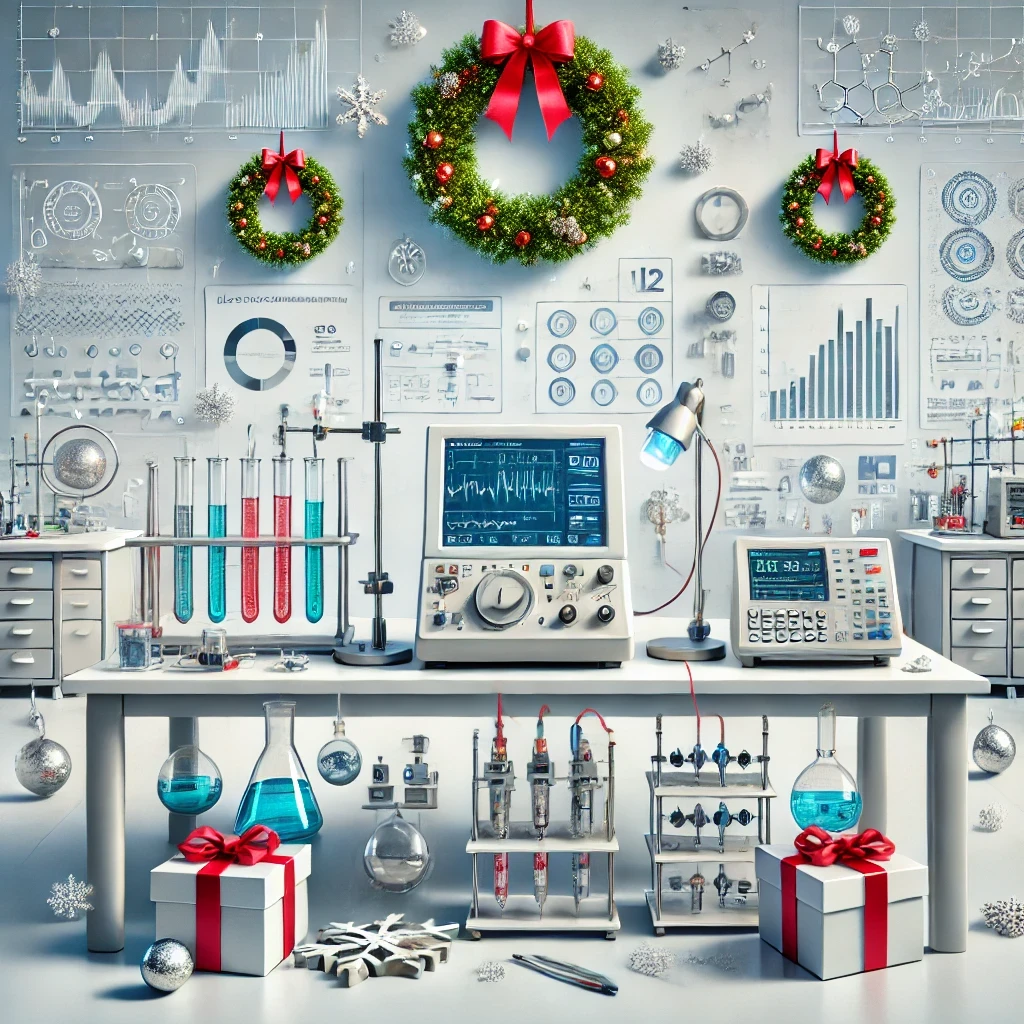
12 Days of Electrochemical Testing
To celebrate the holiday season and the re-release of Ionworks Studio, we featured "12 (business) days of electrochemical testing". Each day we pick a test, give a little bit of information about it, and show you how to run it in Ionworks. 🔋 🎄
Dec 17, 2024
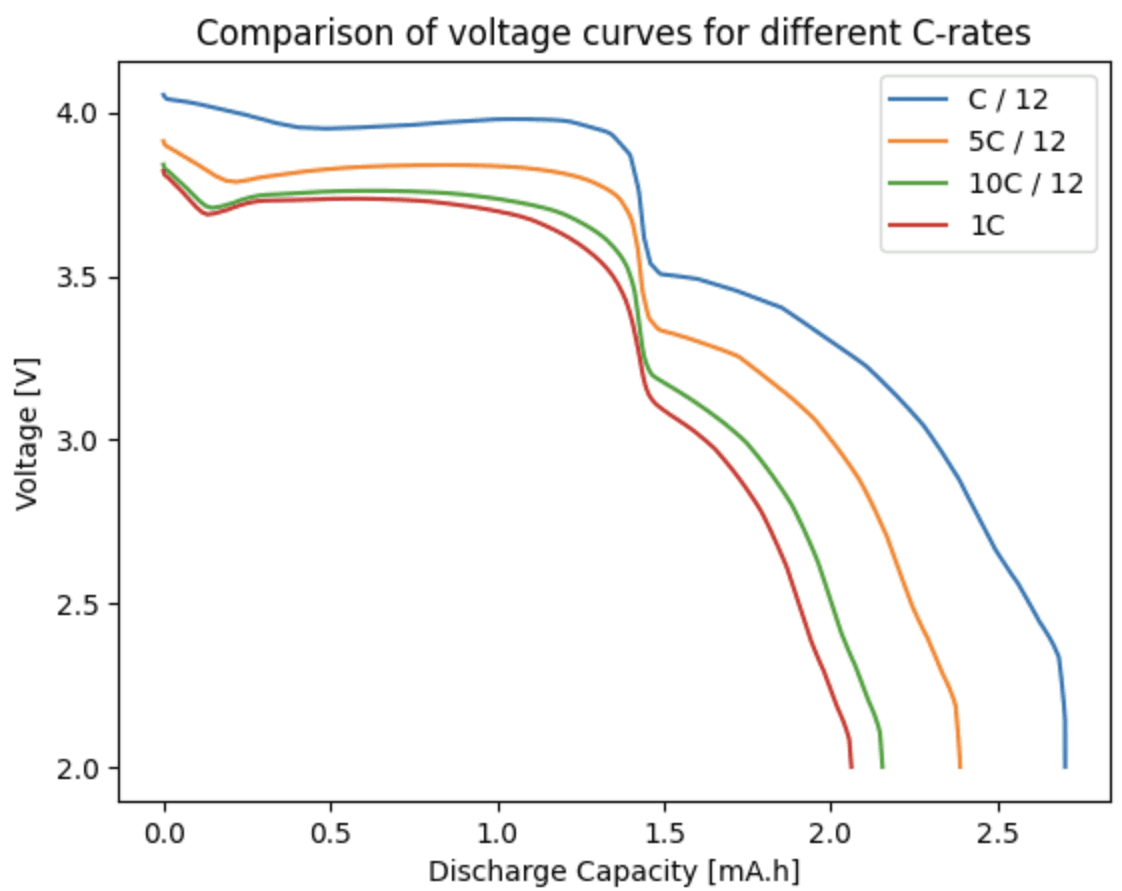
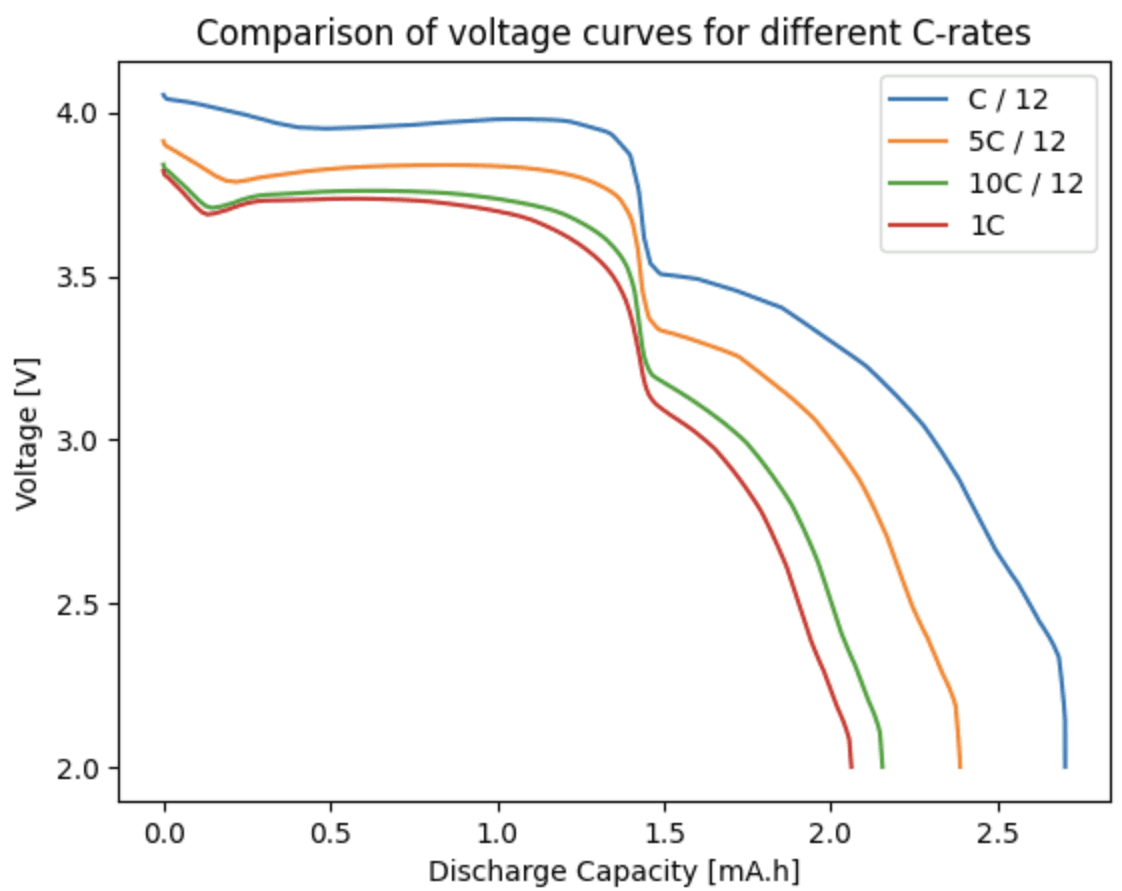
Sodium Ion Battery Model now available in PyBaMM!
This blog post explores the history of SIBs, the intricacies of the new PyBaMM model, and the exciting possibilities it unlocks for the future of energy storage.
Nov 12, 2024
Run your first virtual battery test today
Simulate, iterate, and validate your cell configurations with no lab time required.
Ionworks Technologies Inc. All rights reserved.
Run your first virtual battery test today
Simulate, iterate, and validate your cell configurations with no lab time required.
Run your first virtual battery test today
Simulate, iterate, and validate your cell configurations with no lab time required.
Ionworks Technologies Inc. All rights reserved.
Run your first virtual battery test today
Simulate, iterate, and validate your cell configurations with no lab time required.
Ionworks Technologies Inc. All rights reserved.